Improving on Gradient Descent Algorithm
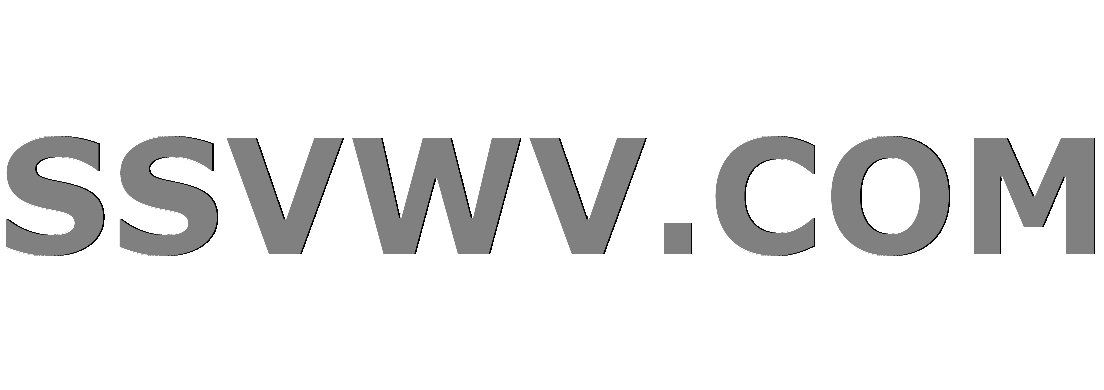
Multi tool use
Clash Royale CLAN TAG#URR8PPP
.everyoneloves__top-leaderboard:empty,.everyoneloves__mid-leaderboard:empty margin-bottom:0;
up vote
4
down vote
favorite
I have tried to solve the problem 607 in Project Euler using the Gradient Descent Algorithm. While I’m getting the answer right I’m not sure if I have used the gradient algorithm correctly or coded it efficiently in Python. I’ll appreciate any guidance or suggestions to improve.
#!/usr/bin/env python3
# -*- coding: utf-8 -*-
"""
Created on Thu Jan 11 18:48:41 2018
"""
# Gradient Descent Algorithm
import numpy as np
import time
import random
def duration(points): # Duration as the objective function
speed = [ 10, 9, 8, 7, 6, 5,10 ]
duration=0
for i in range(0,len(speed)):
distance = ((points[i+1,0]-points[i,0])**2 + (points[i+1,1]-points[i,1])**2)**0.5
duration += distance / speed[i]
return duration
def gradient_function(points): # Gradient: Partial differential of duration function f(x) wrt x
speed = [ 10, 9, 8, 7, 6, 5,10 ]
gradient = 0 #np.zeros((8,2))
for i in range(0,len(speed)):
gradient += ((points[i+1,0]-points[i,0])/(speed[i]*((points[i+1,0]-points[i,0])**2 + (points[i+1,1]-points[i,1])**2)**0.5))
return gradient
# Generate randon number between -1 and +1
def myrand():
seed = random.random() * 2 - 1
return seed
# Compute x = x0-delta*gradient
def gradient_descent(points,delta):
old_Duration = duration(points)
new_Duration = old_Duration - delta * gradient_function(points)
if myrand() > 0:
delta = -delta
id = int(random.random()*6)+1
points[id,0] += delta
new_Duration = old_Duration - (1+ delta)*delta * gradient_function(points)
if (duration(points) >= old_Duration):
points[id,0] -= delta
new_Duration = old_Duration - (1-delta)*delta * gradient_function(points)
return new_Duration
# Floating Point Range Function
def frange(start, stop, step):
i = start
while i >= stop:
yield i
i /= step
return step
# Calculate the Minimum Days
def min_days():
normal_terrain = ((100 / np.sqrt(2) - 50) / 2)
marsh = 10.
ini_xy = [ 0., normal_terrain, marsh, marsh, marsh, marsh, marsh, normal_terrain ]
y_coords =
x_coords =
k = 0
for i in range(0,len(ini_xy)):
k += ini_xy[i]
y_coords.append(k)
x_coords.append(k)
points = (np.stack((x_coords,y_coords), axis=-1))
for delta in frange(1e-2, 1e-10, 10):
for i in range(10000):
gradient_descent(points,delta)
min_days = gradient_descent(points,delta)
return min_days
start = time.time()
answer = min_days()
elapsed = time.time() - start
print("nThe Minimum Days is %s found in %s seconds" % (answer,elapsed))
python algorithm
migrated from stackoverflow.com Jan 12 at 15:48
This question came from our site for professional and enthusiast programmers.
add a comment |Â
up vote
4
down vote
favorite
I have tried to solve the problem 607 in Project Euler using the Gradient Descent Algorithm. While I’m getting the answer right I’m not sure if I have used the gradient algorithm correctly or coded it efficiently in Python. I’ll appreciate any guidance or suggestions to improve.
#!/usr/bin/env python3
# -*- coding: utf-8 -*-
"""
Created on Thu Jan 11 18:48:41 2018
"""
# Gradient Descent Algorithm
import numpy as np
import time
import random
def duration(points): # Duration as the objective function
speed = [ 10, 9, 8, 7, 6, 5,10 ]
duration=0
for i in range(0,len(speed)):
distance = ((points[i+1,0]-points[i,0])**2 + (points[i+1,1]-points[i,1])**2)**0.5
duration += distance / speed[i]
return duration
def gradient_function(points): # Gradient: Partial differential of duration function f(x) wrt x
speed = [ 10, 9, 8, 7, 6, 5,10 ]
gradient = 0 #np.zeros((8,2))
for i in range(0,len(speed)):
gradient += ((points[i+1,0]-points[i,0])/(speed[i]*((points[i+1,0]-points[i,0])**2 + (points[i+1,1]-points[i,1])**2)**0.5))
return gradient
# Generate randon number between -1 and +1
def myrand():
seed = random.random() * 2 - 1
return seed
# Compute x = x0-delta*gradient
def gradient_descent(points,delta):
old_Duration = duration(points)
new_Duration = old_Duration - delta * gradient_function(points)
if myrand() > 0:
delta = -delta
id = int(random.random()*6)+1
points[id,0] += delta
new_Duration = old_Duration - (1+ delta)*delta * gradient_function(points)
if (duration(points) >= old_Duration):
points[id,0] -= delta
new_Duration = old_Duration - (1-delta)*delta * gradient_function(points)
return new_Duration
# Floating Point Range Function
def frange(start, stop, step):
i = start
while i >= stop:
yield i
i /= step
return step
# Calculate the Minimum Days
def min_days():
normal_terrain = ((100 / np.sqrt(2) - 50) / 2)
marsh = 10.
ini_xy = [ 0., normal_terrain, marsh, marsh, marsh, marsh, marsh, normal_terrain ]
y_coords =
x_coords =
k = 0
for i in range(0,len(ini_xy)):
k += ini_xy[i]
y_coords.append(k)
x_coords.append(k)
points = (np.stack((x_coords,y_coords), axis=-1))
for delta in frange(1e-2, 1e-10, 10):
for i in range(10000):
gradient_descent(points,delta)
min_days = gradient_descent(points,delta)
return min_days
start = time.time()
answer = min_days()
elapsed = time.time() - start
print("nThe Minimum Days is %s found in %s seconds" % (answer,elapsed))
python algorithm
migrated from stackoverflow.com Jan 12 at 15:48
This question came from our site for professional and enthusiast programmers.
add a comment |Â
up vote
4
down vote
favorite
up vote
4
down vote
favorite
I have tried to solve the problem 607 in Project Euler using the Gradient Descent Algorithm. While I’m getting the answer right I’m not sure if I have used the gradient algorithm correctly or coded it efficiently in Python. I’ll appreciate any guidance or suggestions to improve.
#!/usr/bin/env python3
# -*- coding: utf-8 -*-
"""
Created on Thu Jan 11 18:48:41 2018
"""
# Gradient Descent Algorithm
import numpy as np
import time
import random
def duration(points): # Duration as the objective function
speed = [ 10, 9, 8, 7, 6, 5,10 ]
duration=0
for i in range(0,len(speed)):
distance = ((points[i+1,0]-points[i,0])**2 + (points[i+1,1]-points[i,1])**2)**0.5
duration += distance / speed[i]
return duration
def gradient_function(points): # Gradient: Partial differential of duration function f(x) wrt x
speed = [ 10, 9, 8, 7, 6, 5,10 ]
gradient = 0 #np.zeros((8,2))
for i in range(0,len(speed)):
gradient += ((points[i+1,0]-points[i,0])/(speed[i]*((points[i+1,0]-points[i,0])**2 + (points[i+1,1]-points[i,1])**2)**0.5))
return gradient
# Generate randon number between -1 and +1
def myrand():
seed = random.random() * 2 - 1
return seed
# Compute x = x0-delta*gradient
def gradient_descent(points,delta):
old_Duration = duration(points)
new_Duration = old_Duration - delta * gradient_function(points)
if myrand() > 0:
delta = -delta
id = int(random.random()*6)+1
points[id,0] += delta
new_Duration = old_Duration - (1+ delta)*delta * gradient_function(points)
if (duration(points) >= old_Duration):
points[id,0] -= delta
new_Duration = old_Duration - (1-delta)*delta * gradient_function(points)
return new_Duration
# Floating Point Range Function
def frange(start, stop, step):
i = start
while i >= stop:
yield i
i /= step
return step
# Calculate the Minimum Days
def min_days():
normal_terrain = ((100 / np.sqrt(2) - 50) / 2)
marsh = 10.
ini_xy = [ 0., normal_terrain, marsh, marsh, marsh, marsh, marsh, normal_terrain ]
y_coords =
x_coords =
k = 0
for i in range(0,len(ini_xy)):
k += ini_xy[i]
y_coords.append(k)
x_coords.append(k)
points = (np.stack((x_coords,y_coords), axis=-1))
for delta in frange(1e-2, 1e-10, 10):
for i in range(10000):
gradient_descent(points,delta)
min_days = gradient_descent(points,delta)
return min_days
start = time.time()
answer = min_days()
elapsed = time.time() - start
print("nThe Minimum Days is %s found in %s seconds" % (answer,elapsed))
python algorithm
I have tried to solve the problem 607 in Project Euler using the Gradient Descent Algorithm. While I’m getting the answer right I’m not sure if I have used the gradient algorithm correctly or coded it efficiently in Python. I’ll appreciate any guidance or suggestions to improve.
#!/usr/bin/env python3
# -*- coding: utf-8 -*-
"""
Created on Thu Jan 11 18:48:41 2018
"""
# Gradient Descent Algorithm
import numpy as np
import time
import random
def duration(points): # Duration as the objective function
speed = [ 10, 9, 8, 7, 6, 5,10 ]
duration=0
for i in range(0,len(speed)):
distance = ((points[i+1,0]-points[i,0])**2 + (points[i+1,1]-points[i,1])**2)**0.5
duration += distance / speed[i]
return duration
def gradient_function(points): # Gradient: Partial differential of duration function f(x) wrt x
speed = [ 10, 9, 8, 7, 6, 5,10 ]
gradient = 0 #np.zeros((8,2))
for i in range(0,len(speed)):
gradient += ((points[i+1,0]-points[i,0])/(speed[i]*((points[i+1,0]-points[i,0])**2 + (points[i+1,1]-points[i,1])**2)**0.5))
return gradient
# Generate randon number between -1 and +1
def myrand():
seed = random.random() * 2 - 1
return seed
# Compute x = x0-delta*gradient
def gradient_descent(points,delta):
old_Duration = duration(points)
new_Duration = old_Duration - delta * gradient_function(points)
if myrand() > 0:
delta = -delta
id = int(random.random()*6)+1
points[id,0] += delta
new_Duration = old_Duration - (1+ delta)*delta * gradient_function(points)
if (duration(points) >= old_Duration):
points[id,0] -= delta
new_Duration = old_Duration - (1-delta)*delta * gradient_function(points)
return new_Duration
# Floating Point Range Function
def frange(start, stop, step):
i = start
while i >= stop:
yield i
i /= step
return step
# Calculate the Minimum Days
def min_days():
normal_terrain = ((100 / np.sqrt(2) - 50) / 2)
marsh = 10.
ini_xy = [ 0., normal_terrain, marsh, marsh, marsh, marsh, marsh, normal_terrain ]
y_coords =
x_coords =
k = 0
for i in range(0,len(ini_xy)):
k += ini_xy[i]
y_coords.append(k)
x_coords.append(k)
points = (np.stack((x_coords,y_coords), axis=-1))
for delta in frange(1e-2, 1e-10, 10):
for i in range(10000):
gradient_descent(points,delta)
min_days = gradient_descent(points,delta)
return min_days
start = time.time()
answer = min_days()
elapsed = time.time() - start
print("nThe Minimum Days is %s found in %s seconds" % (answer,elapsed))
python algorithm
edited Jan 12 at 16:02


Billal BEGUERADJ
1
1
asked Jan 12 at 13:08
Srini
migrated from stackoverflow.com Jan 12 at 15:48
This question came from our site for professional and enthusiast programmers.
migrated from stackoverflow.com Jan 12 at 15:48
This question came from our site for professional and enthusiast programmers.
add a comment |Â
add a comment |Â
1 Answer
1
active
oldest
votes
up vote
2
down vote
For Python 3.x you do not need to specify the encoding type you chose because it chips by default with UTF-8. So you can safely remove the directive:
# -*- coding: utf-8 -*-
You should use timeit over time because the first one is more accurate.
As per PEP 8, you should leave 2 blank lines between the last import statement and the start of your code.
Better if you run this module by putting the guard
if __name__ == "__main__"
- The inline comments you used would be better used as docstrings instead.
add a comment |Â
1 Answer
1
active
oldest
votes
1 Answer
1
active
oldest
votes
active
oldest
votes
active
oldest
votes
up vote
2
down vote
For Python 3.x you do not need to specify the encoding type you chose because it chips by default with UTF-8. So you can safely remove the directive:
# -*- coding: utf-8 -*-
You should use timeit over time because the first one is more accurate.
As per PEP 8, you should leave 2 blank lines between the last import statement and the start of your code.
Better if you run this module by putting the guard
if __name__ == "__main__"
- The inline comments you used would be better used as docstrings instead.
add a comment |Â
up vote
2
down vote
For Python 3.x you do not need to specify the encoding type you chose because it chips by default with UTF-8. So you can safely remove the directive:
# -*- coding: utf-8 -*-
You should use timeit over time because the first one is more accurate.
As per PEP 8, you should leave 2 blank lines between the last import statement and the start of your code.
Better if you run this module by putting the guard
if __name__ == "__main__"
- The inline comments you used would be better used as docstrings instead.
add a comment |Â
up vote
2
down vote
up vote
2
down vote
For Python 3.x you do not need to specify the encoding type you chose because it chips by default with UTF-8. So you can safely remove the directive:
# -*- coding: utf-8 -*-
You should use timeit over time because the first one is more accurate.
As per PEP 8, you should leave 2 blank lines between the last import statement and the start of your code.
Better if you run this module by putting the guard
if __name__ == "__main__"
- The inline comments you used would be better used as docstrings instead.
For Python 3.x you do not need to specify the encoding type you chose because it chips by default with UTF-8. So you can safely remove the directive:
# -*- coding: utf-8 -*-
You should use timeit over time because the first one is more accurate.
As per PEP 8, you should leave 2 blank lines between the last import statement and the start of your code.
Better if you run this module by putting the guard
if __name__ == "__main__"
- The inline comments you used would be better used as docstrings instead.
answered Jan 12 at 15:59


Billal BEGUERADJ
1
1
add a comment |Â
add a comment |Â
Sign up or log in
StackExchange.ready(function ()
StackExchange.helpers.onClickDraftSave('#login-link');
);
Sign up using Google
Sign up using Facebook
Sign up using Email and Password
Post as a guest
StackExchange.ready(
function ()
StackExchange.openid.initPostLogin('.new-post-login', 'https%3a%2f%2fcodereview.stackexchange.com%2fquestions%2f184963%2fimproving-on-gradient-descent-algorithm%23new-answer', 'question_page');
);
Post as a guest
Sign up or log in
StackExchange.ready(function ()
StackExchange.helpers.onClickDraftSave('#login-link');
);
Sign up using Google
Sign up using Facebook
Sign up using Email and Password
Post as a guest
Sign up or log in
StackExchange.ready(function ()
StackExchange.helpers.onClickDraftSave('#login-link');
);
Sign up using Google
Sign up using Facebook
Sign up using Email and Password
Post as a guest
Sign up or log in
StackExchange.ready(function ()
StackExchange.helpers.onClickDraftSave('#login-link');
);
Sign up using Google
Sign up using Facebook
Sign up using Email and Password
Sign up using Google
Sign up using Facebook
Sign up using Email and Password